Researchers created a motion dataset to train autonomous vehicles on how blind individuals move
Photo licensed by Adobe Stock
the_post_thumbnail_caption(); ?>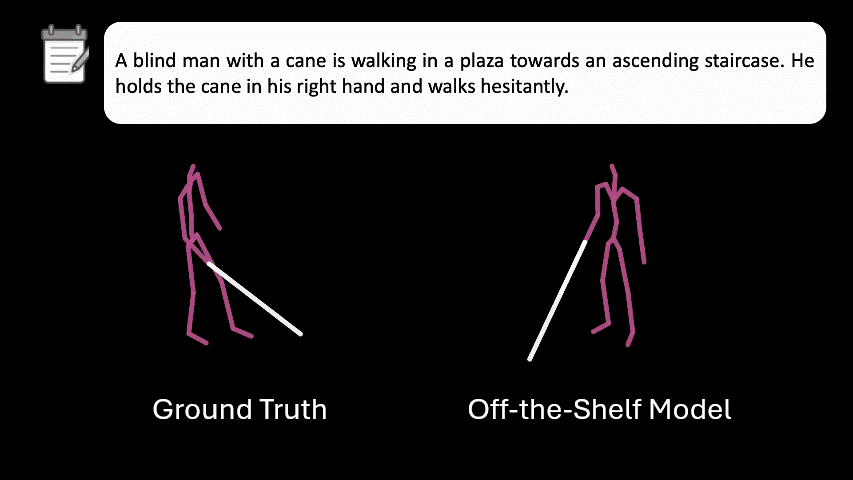
During the Tokyo 2020 Paralympic Games, one of Toyota’s autonomous vehicles, part of its e-Palette fleet designed for the Games, collided with a blind athlete in the Olympic Village while crossing a junction at low speed. The incident highlights the need for autonomous vehicles to be more aware of how blind pedestrians move through streets. University of Maryland College of Information and UMIACS Associate Professor Hernisa Kacorri and Boston University College of Engineering Assistant Professor Eshed Ohn-Bar, along with graduate students Hee Jae Kim, Kathakoli Sengupta, and Masaki Kuribayashi, created BlindWays, a novel dataset with real-world 3D motion capture data and detailed descriptions of how blind individuals navigate their environment.
“We realized that most datasets and models for understanding human movement only include sighted people,” says Kacorri. This oversight can hinder the ability of autonomous vehicles to safely predict the movements of blind pedestrians, whose behaviors, such as using a cane to feel the curbs or veering, might confuse current models leading to potentially dangerous errors. “Getting these predictions wrong can be a matter of life and death,” she says.
Traditional motion datasets are often collected in controlled indoor environments, where actors reenact movements. However, these setups do not accurately reflect real-life human motion. To ensure the authenticity of BlindWays, researchers employed a wearable motion capture system with 18 sensors to track body and mobility aid movements. “For BlindWays, we wanted the data to be as realistic and natural as possible,” says Ohn-Bar.
Designing Real-World Challenges
Researchers collaborated with the blind community to ensure routes used for the study accurately captured what blind pedestrians encounter in an urban setting. They designed eight urban routes with real-world challenges such as stairs, uneven pavement, and busy sidewalks. Blind participants navigated these routes with canes or guide dogs. In addition to the 3D motion data, the researchers collected detailed textual descriptions on how participants moved, interacted with their environment, and their navigation aids.
“We had a team of annotators, including experts in biomechanics, sensorimotor studies, and mobility research, create detailed textual descriptions for each motion in the dataset,” says Kacorri. “These descriptions capture the finer details of how blind participants navigate, like how they use their cane to handle obstacles, their goals, or how confident they are in different situations. Think of it as a quick summary of their movements. These descriptions are also crucial for training models that combine language and motion. By tweaking the text input, we can test if the models can accurately simulate realistic motion scenarios for blind pedestrians.”
The results of their research are encouraging. “Training with BlindWays has shown promise, reducing prediction errors by over 80% in some cases and highlighting the importance of representative data,” says Ohn-Bar. “However, challenges remain, especially in high-stakes scenarios like crossing or turning, where errors are still too frequent,” he adds.
Future Collaborations and Applications
To enhance and expand the BlindWays dataset, the researchers plan to collaborate with organizations specializing in disability rights, mobility training, and urban planning. These partnerships aim to diversify participants, locations, and scenarios in the dataset, enriching its comprehensiveness and representativeness.
“BlindWays is just the beginning,” says Kacorri. “AI models can act unpredictably when coming across wheelchair users, people with motor impairments, or those who are neurodivergent. These groups face a higher risk in traffic accidents and are often excluded from current datasets. Beyond individual patterns, each group could present distinct movement characteristics. Capturing this diversity is vital to making systems like self-driving cars, delivery robots, and assistive tools break down rather than reinforce existing social and physical barriers.”
Additionally, the researchers anticipate partnerships with technology developers. “There are exciting possibilities, from raising public awareness about the challenges blind individuals face to personalizing assistive systems based on mobility aid strategies, or even creating more realistic and inclusive animations of blind people in virtual platforms and video games,” says Ohn-Bar.